Who Are The Poor Of India?
Ever since I quit the corporate world, the story I have been telling myself is that I want to work on uplifting the poorest.
I am data-man, or so I would like to think. So, it is essential for me to understand the size and depth of this problem of poverty. And how do you even define poverty? Let alone quantify it?
Over the past three years, I believe I have earned a decent understanding of it. This is an attempt at formalizing that understanding.
First, what is poverty?
Believe it or not, this isn’t straightforward. There are four different ways to look at it:
- Absolute Poverty: This draws an income line across the world and everyone under it is poor and everyone above it is not poor. There are different lines, the most popular being the “extreme poverty” line which is $1.90 / day adjusted for purchasing power parity. Which basically means let’s keep things apples to apples, not apples to moons. Pros and cons here and you can think about that all day.
- Relative Poverty: This arranges people within a certain population according to income, and those that fall in the bottom tranche are considered poor. A good way of understanding this is that while there is no absolute extreme poverty in the U.S., there’s a ton of relative poverty. Relative poverty will almost always exist. Absolute poverty doesn’t need to.
- Multidimensional Poverty: This is my preferred definition. It is based on the tenet that poverty is more than just a lack of money — it is a lack of access to healthcare, education and a decent standard of living. It just falls short of including dignity, but dignity is something even the Gods won’t be able to quantify. Intuitively, this increases the total number of poor because it increases that basic standard we aspire to for humanity.
- Hunger: If you’re absolutely poor, you’re probably hungry. So, sometimes, looking at the hunger index is also a good way of gauging pain. Sounds morbid, but it is easier to quantify.
And these definitions are just skimming the surface of the ocean that is measuring injustice. There are also recall methods, survey nuances, time-sensitivity issues, and other permutations that give data-abusers enough ammo to craft any story that fits their narrative.
I’m going to avoid doing that.
Okay enough jargon, so how many people in India are poor then?
You guessed right; there are many different estimates for this as well. But I promise I’ll end this section with somewhat of a reasonable number for us to anchor to.
Meanwhile, here is a bunch of poverty estimates for India that you’ll see flying around in the public:
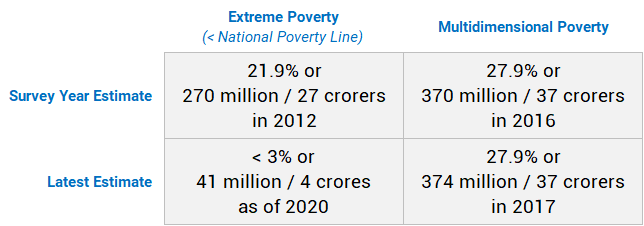
Image by Author, Sources: 2012 Extreme Poverty, 2020 Extreme Poverty, Multidimensional Poverty
It’s important to consider what the numbers were during the survey year because that’s when data is (or should be) the cleanest. Estimates based off that add more assumptions to an assumption-laden world, which only contaminates the truth.
For instance, I don’t fully believe World Poverty Clock’s assumption that India has only 40 million extremely poor people right now. Either way, I don’t think looking at extreme poverty is the best way of looking at specific country’s poverty — it’s singular, and poverty is not singular. So, let’s drop that and progress.
Multidimensional poverty on the other hand, is hands-down the best way to look at poverty based on what’s out there. It was developed by Oxford University in 2010 so there’s some credibility for you. This metric is hard to calculate but not impossible. Enter UNDP. They have been nice enough to calculate and aggregate this for us.
Ahh, I’m finally getting to the point.
In India, in 2017, 28% or 374 million were multidimensionally poor. I did my own math from an incredible human development dataset from 2012 using a similar thought process, and my estimate was ~34% for back then. So, taking all that into consideration, and the fact that India has been growing economically (at least pre-COVID), I think it is fair to assume that today, India has a multidimensional poverty rate of 20%-25%. Extrapolating that to current population estimates (2020), that’s somewhere between 274 million and 342 million people.
To make it a little easier, let’s settle for a number somewhere in the middle which conveniently comes to 300 million people that makes up ~22% of India’s population.
There, as I promised, a reasonable number we can anchor ourselves to.
Fine, so who are the poor?
It’s now time to get to the meat of things. If you’re familiar with the social impact space, none of the above should have been bombastically revealing. The following though? Maybe.
The question that was grappling my mind (and my heart) is who exactly are the poor? And not just from a meta, philosophical perspective, but from an actionable, data-driven perspective.
Farmers, construction workers, waste-pickers, Dalits — that’s what comes to mind right off the bat, but how many of them? By how much? Who else? From what perspective are they poor — health, education, income? And what about security guards and dhobis and coolies? Are they poor? Not all of them, obviously, but how many?
So yes, if work is the center of sustenance of life, I was curious what type of workers fell under the landscape of the poor in India.
I happened upon an incredible human development survey from 2012 that had data at an individual and a household level for over 40,000 households and over 200,000 individuals. It was representative and has been used by many impact strongholds including the International Labour Organization as recently as 2018. Credibility.
I isolated the 50,000 individuals who were mostly working, cleaned up some of the occupation data, mapped it, calculated a reasonable version of multidimensional poverty based on the data that was available, and voila! Here is how I answered those grappling questions of the head (and the heart).
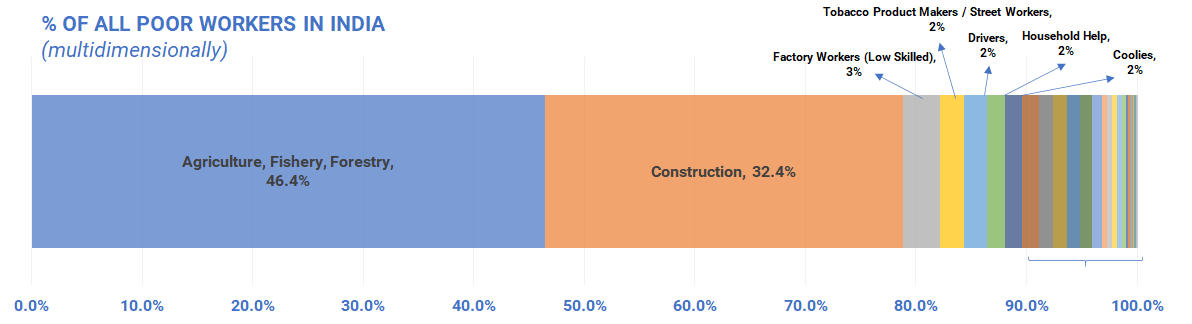
Image by Author, Based on 2012 India Human Development Survey (IHDS)
No surprises here. ~79% of all poor workers in India (62 million) work either in agriculture or construction. Low skilled factory workers come third at 3%, which is substantially lower. The rest are 2% or less. The small revelation for me was the tobacco product makers (beedi-makers) at 2.2% or almost 2 million. They earn the lowest on average and almost a third of all of them are multidimensionally poor. Interesting cognitive dissonance here: tobacco is bad for you but what happens to the millions of people who make a (poor) living out of making tobacco products?
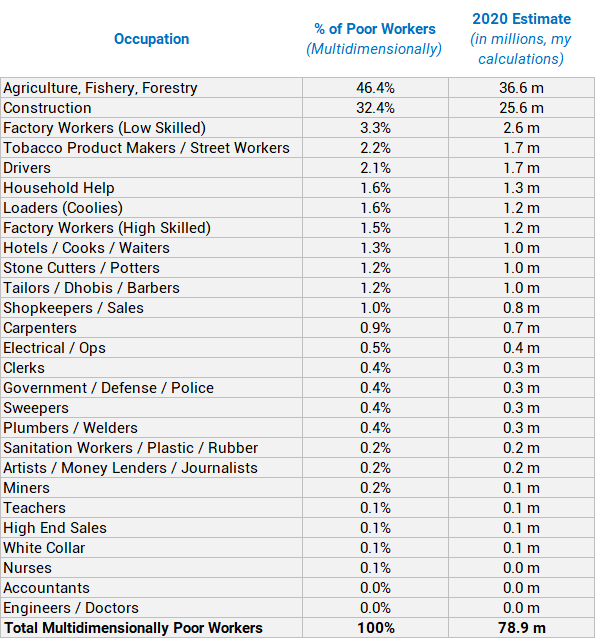
Image by Author, Based on 2012 India Human Development Survey (IHDS)
We now know that most of the poor are farmers and construction workers. But they also constitute the largest proportion of all workers in general. So what percentage of farmers, and construction workers and drivers are poor?
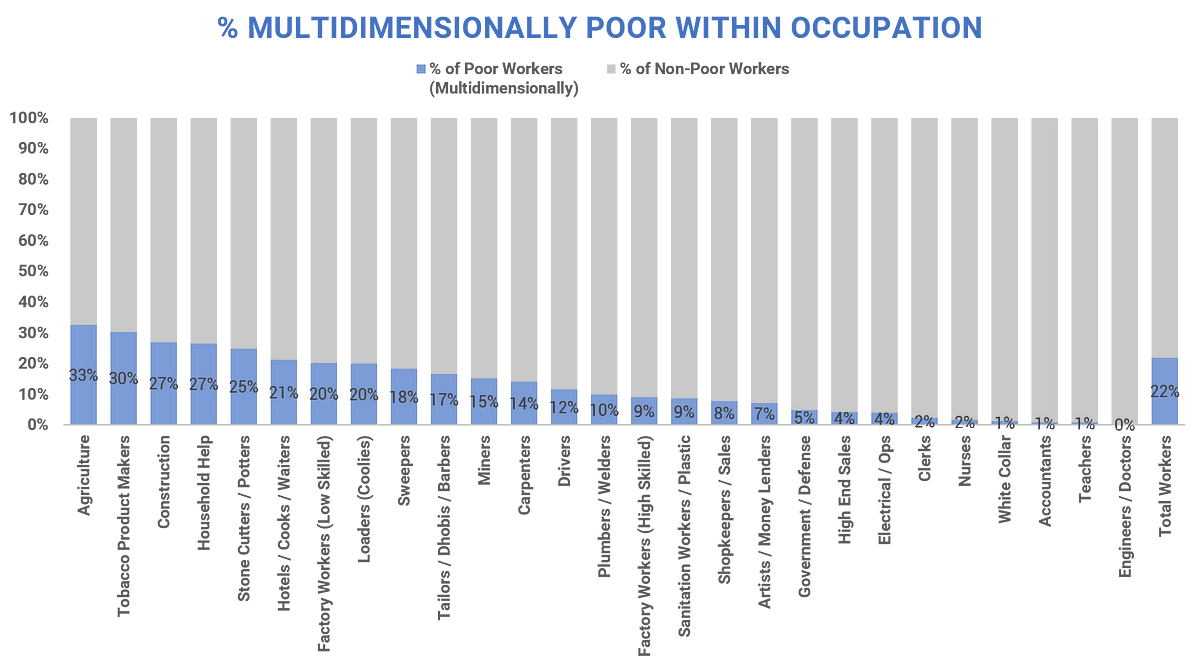
Image by Author, Based on 2012 India Human Development Survey (IHDS) and my calculations
Almost a third of all agricultural workers are poor. Construction workers are also above the average, and so are tobacco product makers, household help and stone cutters. And remember, these folks are not just poor from an economic perspective, they are also less educated and much more at risk with their health.
Modified HDI
Inspired by UNDP’s Human Development Index (HDI), I created a morphed / modified version of it for the various types of workers of India. Why morphed? Well because I was limited by the information available by worker in the data set I was using. Enough excuses, enough disclaimers, it is still helpful, albeit not telling us anything spectacularly new.
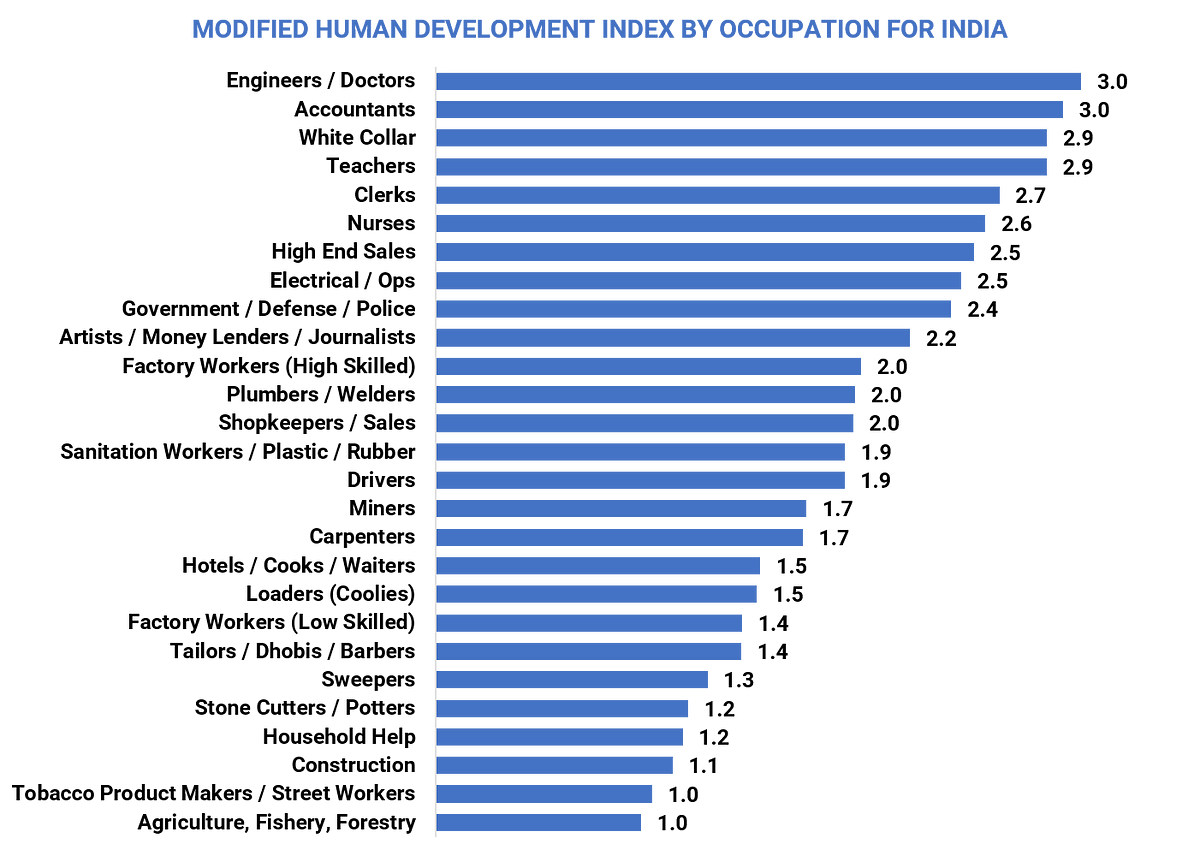
Similar story — farmers, tobacco makers, construction workers, maids and stone cutters live a disdainful life.
One of the morphs I sizzled into this modified HDI was the inclusion of a social status index. This is a tad bit controversial and it’s pretty sad that I even have to include something like this, but in India, it’s just not advantageous being born into a lower caste or a marginalized minority. Adding that dimension into the mix pulls the occupation group of sweepers lower down. Most sweepers and sanitation workers (~60%) are dalits (low caste folks). Most stone cutters are also dalits and many are adivasis (protected tribes). This shouldn’t make a difference, but it does, and not for the right reasons.
Anything else?
Look, this is still far from perfect. Just because many farmers and constructions workers are poor, doesn’t mean that they are the poorest. Just because some people have money and healthcare and education, doesn’t mean they live happy and wholesome lives. Quantifying dignity, happiness, pain and injustice are hard if not impossible.
But that doesn’t mean we can’t try. And that is not an excuse to not do anything about it.
So now what?
Let’s get at it. As effectively and quickly as we can, while still ensuring we seek systemic solutions that solve this problem for the long run. I don’t think we need a quick fix per se, I think we need long-term fix sooner rather than later. We have made enough progress today to envision a world where multidimensional poverty does not exist.
I essentially did this analysis for myself. I will start a social enterprise focusing on this very issue, and wanted to understand it better. And despite breaking the problem down, I am still battling through other dimensions that are hard to reconcile.
If you have any epiphanies, please decorate me with them.
A note on the sources for this post:
The base for the majority of this analysis is the micro-data of the India Human Development Survey: Desai, Sonalde, Reeve Vanneman and National Council of Applied Economic Research, New Delhi. India Human Development Survey-II (IHDS-II), 2011–12. ICPSR36151-v2. Ann Arbor, MI: Inter-university Consortium for Political and Social Research [distributor], 2015–07–31. http://doi.org/10.3886/ICPSR36151.v2
I created and cleaned some of the data to make it more sensible.
I have cited sources for all one-off data where I have used them.
Any questions / concerns, please do not hesitate to reach out. Am a believer in full transparency.
Blah
I woke up a little dejected this morning. With a sense of futility and fatigue that eclipses the extremes of physical and mental burnout. The best word for it is probably blah.
I saw the Barstool founder eloquently rant away about the totalitarian lockdown. And then there was banter between him and Musk.
I then read about how Russian doctors are dropping like flies — diseased, either mentally or physically, while somehow falling through windows.
My morning coffee ritual is a conversation with my uncle who I am locked in with. He talked about how one of my grand aunts was stuck in Singapore for a couple of months. She just got jetted into India. And is now held in quarantine for fourteen days. She will be tested, and then “released.” A weird kind of prison. He uncharacteristically philosophized well: “What has life become?”
I thought about my two phone conversations with migrant workers yesterday. Both were organizing large-ish groups of people — family, friends, ten, twenty, forty people. They had just been bussed from Maharashtra to Madhya Pradesh. They had arrived so close to their home yet were forty kilometers away.
They had no money for the last mile, to get them to their homes. It was a weird kind of torture. They needed a few thousand rupees to organize private transport to get them home. Otherwise, they would have two options: walk the forty kilometers, or stay there for the night and figure it out in the morning.
A friend who has been hustling to mitigate this migrant tragedy called frantically to see if I could sort them out quickly. Bless bank transfers in India because the solution was almost instant. After, one of them who I spoke to on the phone said: “You’ve become Godlike for us now.” And I was swept over with shame.
I thought about my introverted, genius uncle who has found a virtual extroversion through LinkedIn. With almost 150,000 followers, he has a voice. He has been extremely anti-lockdown, and not shy about it. He is one the most well-read and informed people I know, and cited evidence and reason to support his claim. He was swiftly banned by LinkedIn for opposing prevailing “best” practices. He has a following of both fans and haters. He has made it.
I thought about the two camps that are growing around a line of control that seems completely out of control. On one side you have the elites yelling “shut everything down”, “lives matter more than economy”, “black swan!”, etc. And then you have the other side where the elites are also yelling “free us!”, “the economy matters and might take more lives”, “<0.5% mortality rate”, “this is an invasion on our freedom”, etc.
It is just so hard to know which camp makes more sense. The answer always lies somewhere in the middle. But even the middle can be slightly skewed one way or the other. What way then? And does it even matter what I think?
And this brings to mind Haidt’s wisdom— once we put our opinion out there in the public — on Twitter, on LinkedIn — we defend it religiously, sometimes beyond reason. Our pride gets in the way of us changing our mind. A fear that we might be framed hypocrites. Because social status is everything. And never has it been easier to gain it. And lose it. So, once you gain it, does it not behoove you to try and keep it? At any cost?
The kicker is that we are not necessarily aware of this. The smarter you are, the better you are at deluding yourself to believe your stance. The better you are at finding evidence that supports your claim. So, what is balance then?
If you are not spared for being wrong, there is no incentive to change your mind, or chase truth. I wonder if my genius uncle, Fauci, Musk, Taleb, Naval will ever change their mind. There is so much to lose.
While us privileged few contemplate what is the best course of action, or try new recipes, and spend more time with our children, or fight the moral dilemma about wanting to spend less time with our children; there is this whole other distant, less fortunate world out there that is only getting more distant. How does our privilege impact our moral obligation?
Never have I felt so strongly about my moral obligation — I am lucky to sleep eight hours every night, in air conditioning, eating delicious food during this unprecedented modern tragedy. All the while, there are billions living their worst days. How can I not try and do something about it?
Excerpts From Sangamner
Thursday, March 5th, 2020–1:23pm, Sangamner, India.
All kinds of humbled right now.
It is my second day here in Sangamner, and it feels like so much has already happened. I have been in India for over two months now and it’s been a little surreal, a little slow, a little lost, a little uncertain. I have been a little sick throughout, and it feels like a little bit of a lot of the world is falling ill along with me as the Corona Virus sweeps fear across the masses. It’s all a bit strange right now in the world, and yet somehow, all I can find is a little bit of optimism.
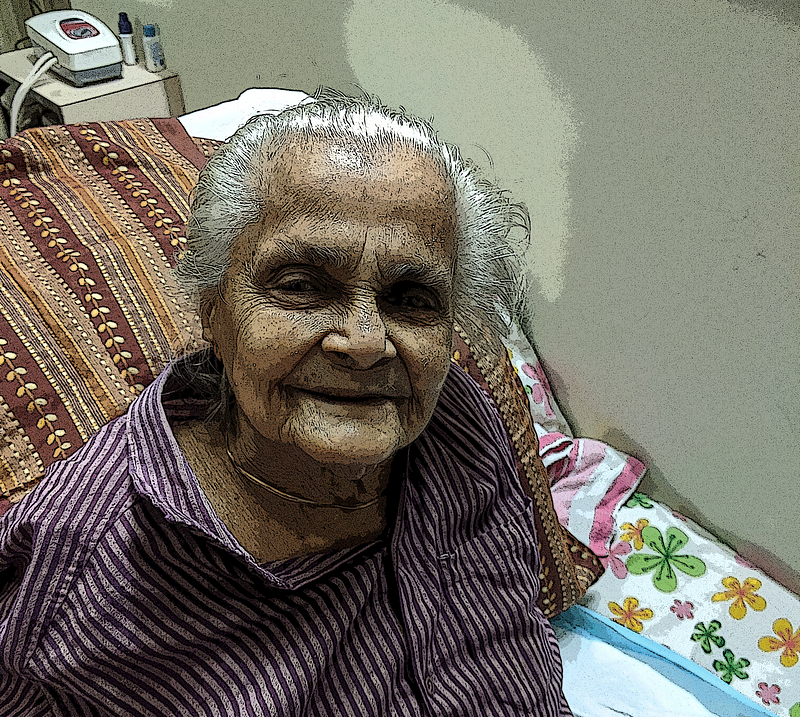
My ninety-three-year-old grandma is lying in front me. She is moaning softly, meekly, sporadically. She can barely move. It’s like she is crying to herself, but she yearns God. It seems like she is taking God’s name in vain, but the truth is, she is taking God’s name with a sense of futility that only death beckons. She has seen better days.
Yesterday, she fell off her bed. She had been doing better, and all it took was a lapse in judgment, a moment of over-confidence, and she came crashing down. I wasn’t there, but I was told. She fell onto her nightly helper, who also hurt herself. My grandma has gotten a bit heavy — too many laddoos that almost seem like compensation for her dearth of joy, the lack of purpose that old age brings.
My eldest uncle and aunt, Babaji and Maa, are God-sent. A different breed with a different, elevated level of integrity that would humble even the wisest. As I grow old, I am learning that wisdom, more often than not, lies in action, not in words.
They were supposed to be going to Kenya to enjoy the wilderness of where it all began. But the fear amassed among the people of the world cancelled their escape. The Corona Virus might be only as dangerous as the common flu, but the fear of the unknown that it elicits has already brought the world to its knees. And it’s only the beginning. It’s as if one of our greatest unifiers, fear, might also be our greatest downfall. Or a just a brutal reset as prescribed by the scathed mother nature.
The disease didn’t scare away Babaji and Maa. Babaji said to me — death is certain, it’ll find us whether we are in Kenya or not. But instead, societal norms shackled their will. The couple they were supposed to go with buckled under pressure and Babaji and Maa caved into having their trip postponed. I urged them to go without the other couple — everything was already booked and paid for — but they said quietly, “Aise nahi hota hain” — this is not how it works. That’s how India is sometimes — culture becomes reason.
And just when their trip was getting cancelled, my grandma fell. It seemed like a sign, and it was enough to rationalize that they had to stay.
My grandma doesn’t fully recognize me anymore. I am a familiar face at best, and my name, a lost memory, confused constantly with another cousin. But it doesn’t matter. Nothing really matters to her anymore and not as a function of her arrogance, but as a function of her pain. Of boredom. Of an inability to contribute. It’s like she is looking for peace and is starting to realize that only the end will bring it to her.
It’s such a stark scene. My grandma, the pillar of our family always standing strong, the woman who worked three jobs to give her children a chance to succeed, is now reduced to a weaker version, humbled by the humanity that science explains — everything depreciates. Almost on cue, she turns to me and says, “Where do I go from here?”
My grandma is in pain. She can’t really move, and is stuck in bed all day, all the while her body and her mind yearns to move and do and be. She hates that we have to take care of her. She hates that she wears a diaper. She asks me if she is bothering me because I have to look over her at times — “is this why you came here?”
Is it even worth it anymore for her? She’s 93. Lived a whole-hearted life, filled with love and growth and accomplishment. Now this dementia, this pain, just seems like throwing the shit she sometimes sleeps in on a beautiful painting she spent all her life drawing. Why ruin it at all in the end?
Old age sucks.
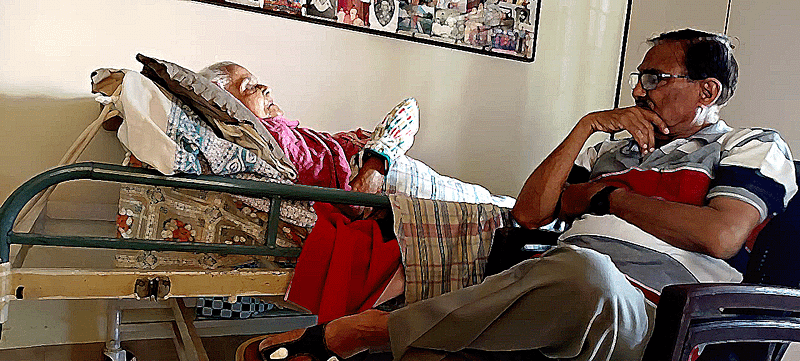
Friday, March 6th, 2020–6:44pm, Sangamner, India.
I wanted to write more yesterday.
Sangamner is different this time around. Not because it has changed, but for once, I have come here with a different lens, a different purpose — not just to visit family and “tolerate” the backwardness of suburban India, but to imbibe what it means to live here. As Dad says, everything is research for me right now. And just like that, everything is different for me even though it’s all the just the same.
Yesterday, it was my Maa’s birthday. On an average day, she is neck-deep busy, advocating for the rights of her clients, taking care of my grandma, and the tens of other things she does. She wakes up at 5 in the morning, leads a two hour session of Yoga for some of the older women of the town, marshals the troops at home afterwards, goes to court, comes back for lunch, goes to court again, comes back for an early dinner, feeds my grandma if she can, goes back to court, comes back, runs errands and then goes to sleep around 11 at night. It all starts again the next morning at 5. This has been in play for the last forty odd years. And yesterday was her birthday.
Her birthday almost made her phone sound like a thriving call centre. It kept ringing with the joy of well wishes. People kept coming over — so many with so much love. There was so much love that quite literally, it was stressing Maa out. It was a testament to the work she does for her community. And it was a testament to how suburban life in India is. Together. Happy.
The door of our house in Sangamner is only locked at night. Or rather, it’s almost always open. People come and go, with and without a heads up, refusing with effusing amounts of politeness any offering but realizing that they must take something sweet or salty, because that’s what they would have done too. There’s respect, admiration and love, and it doesn’t seem fake. It’s born out of being there for each other, and those that give more, get more of it. My Maa and Babaji give with all their heart.
I feel like I have been blinded all this while. I always wondered why Babaji didn’t leave Sangamner for “greener pastures” only to now realize that he already has the greenest. Money really isn’t it. It’s community. And I know this sounds cliched, but when you see it glaring in your face, and the happiness and self-worth it embodies among the people it embraces, you can’t help but be humbled.
Dubai, Bombay, Pune, the urban West, are just not the same, at least the bits I have seen. I lived three years in New York and never met a neighbour let alone got to know them, and I was by no means the exception, I was very much the rule. Relationships are superficial, driven by things like kids sharing the same age and the same nursery, so their parents become required friends. The nuclear family rules, while driving children into depression, as the illusion of chasing money and comfort denies them their innate hunger to be social animals. And social animals not just with a few, but with many - similar in ways, in thought, in history, in culture. It’s the most intelligent that seem the most brainwashed.
Look, I am fortunate to be preaching this. I was blessed to not have to worry about money, and there’s a luxury there that lets you free your mind. And obviously, all those that have money aren’t depressed — they live decent lives, maybe burying their discomfort in delusion, but it can still be worthy. Rather, what I find compelling is how have we have built a society that revolves around money rather than well-being. Money was an important invention, but did greed win? How do we teach our kids to chase purpose and belonging instead? Why does it seem like going abroad is where the gold is? How did we get our basics wrong? Dopamine is the devil.
I started driving classes here because driving in India is like driving in a different world. The horns, the lights, the people have different rules. The driving class isn’t very helpful — it starts late, I only get fifteen minutes because it’s a group class, and the instructor isn’t great. But I get an hour with four young men of Sangamner and how they tackle their lives there.
On my first day, one of them saw me walking back by myself. He stopped me in the middle of the road, and said aren’t you with the Malpani’s? I said yes, and he said, hop on my scooter and I’ll give you a ride. So, like any Sangmaneri would do, I hopped on to his little scooty and he dropped me off home. Now he will pick me up and drop me off every day. I asked Babaji what that was all about. And he said that he is their lawyer — “of course the kid knows who we are”.
That, in a nutshell is Sangmaner. Small. Together. Happy.
Comfort needs to be redefined.
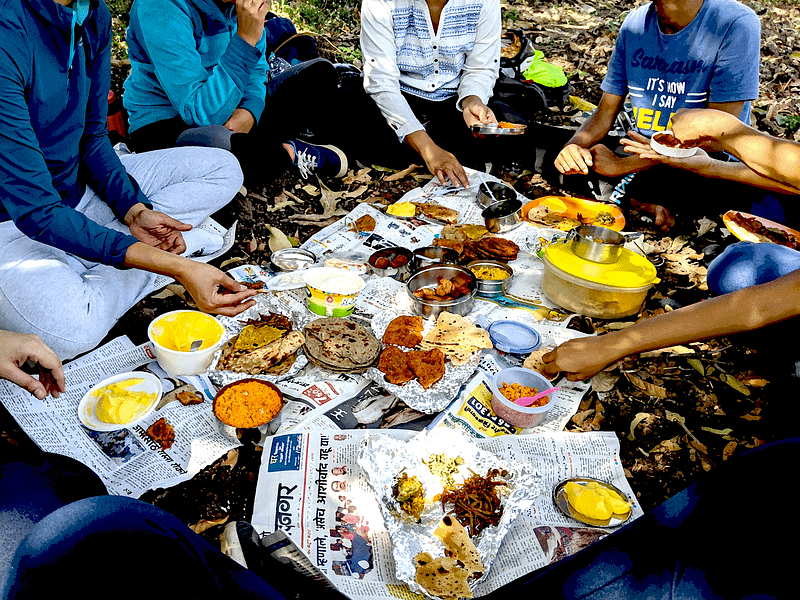
How Can We Impact Well-Being?
Using Machine Learning To Find Out Where To Start
I did an immersive Data Science boot-camp to learn how to better use data to help solve poverty. This dashboard (fancy, huh?) was the output after combining 27 data-sets from 6 different sources, and building an inferential (sometimes predictive) machine learning model using Python, Tableau and good old Excel. Ta dah!
If you’re in the social impact / non-profit space and / or into data, this post outlines how (and why) I went about doing it. It is long and it gets pretty technical, but I hope to make it worth your while.
If you’re like “No, screw that!” and just want to see what the findings are from this, skip right to the end and have a look at the findings and the limitations of this model.
For Coders: Here’s the GitHub link with all the code, the data and the Tableau dashboard file if you’re keen.
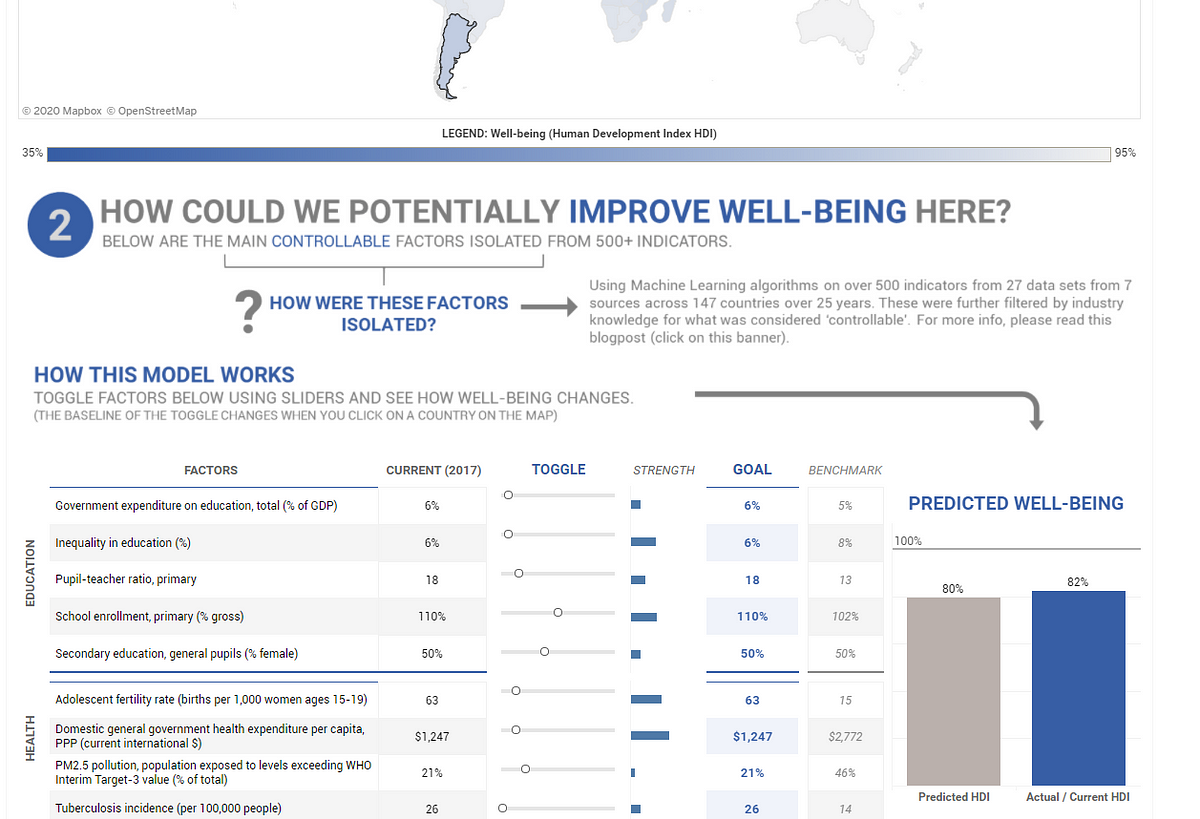
Disclaimers
If you did give the dashboard a gander, some alarm bells might have gone off. That’s fair. No, I’m not claiming to have solved poverty through this dashboard, nowhere close. And there are a lot of assumptions and disclaimers and imperfections that engulf everything about it. That also just happens to be the inherent nature of the impact space — it is really hard to define and measure “impact.”
But that doesn’t mean we can’t try.
The Setup (The “Why”)
Data is the future of decision making, I believe.
This belief drove me to London this past year to do a three-month immersive boot-camp in Data Science through General Assembly.
I already, sort of, know what I am going to do with my life — I want to focus on tackling multidimensional poverty; first through my own social enterprise, and then, if that fails, through whatever makes the most sense.
After spending the last two years working for non-profits, it dawned on me that the impact space, on average, just doesn’t have enough technical expertise. I remember one of the organizations I worked for hired a Fellow and gave him the email address “fellow3@company.org”. I was “financefellow@company.org”.
We have a lot of heart, but we don’t have enough engineers, data scientists, finance experts, graphic designers and other technicians who are integral to operate any type of organizations efficiently.
I did not want to be that cliche. I like data and if I was going to start a social enterprise, I wanted to be at the edge of the latest in tech versus lagging behind.
The Problem
There are over 1.3 billion people who are in multidimensional poverty. What does this “multidimensional” thing mean? Just that — there are multiple dimensions to the concept of poverty — health, education, living standards and the often immeasurable dimension, dignity. This is not as simple as extreme poverty which broad-strokes every person who makes less that $1.90 a day.
How can we tackle this problem as quickly as possible? Poverty has significantly reduced over the last few decades, but there is still a long way to go. So, how can we expedite this process?
There is a lot being done by multilateral organizations (UN, World Bank, IMF), governments and through the contentious way of CSR (still counts though).
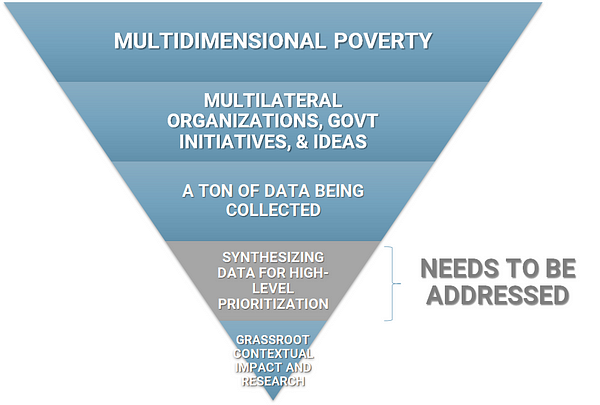
There is also a lot of data being collected by these players — it isn’t necessarily clean or easy to collect, but there is data out there.
My hypothesis though is that there isn’t enough synthesis of this high-level global data. As you can see from the upside down blue triangle above, this is the gap that needs more attention. This is what even the effective altruism movement understands to be true — global priorities research is one of the most pressing problems of the impact space.
So, based on all the global, macro data that is out there, what controllable factors have the highest impact on multidimensional poverty? And can I use machine learning to find any meaningful relationships in the waterfall of data that is out there?
I ran this problem statement by a focus group of key players in the impact space — social entrepreneurs and impact investors — who had some skin in the game. Their gut reaction was, “Wait, what?”
There was a strong belief that the answers are in the field, not hidden in some badly-collected global data. I don’t disagree with that. Whatever the data says, interventions need to be tested locally before large scale implementation, especially because most of the time, lives are at stake.
And then there is the “transferability” of the findings — just because it worked in the past or in Kenya, doesn’t mean it will work today or in India. And I’m not arguing against any of that.
But what if this “badly-collected” global data gave us starting points? What if they gave us hypotheses to test that were better than just intuition or anecdotal evidence? Can we leverage the power of the machine to tell us something that our brains can’t physically calculate?
The Idea
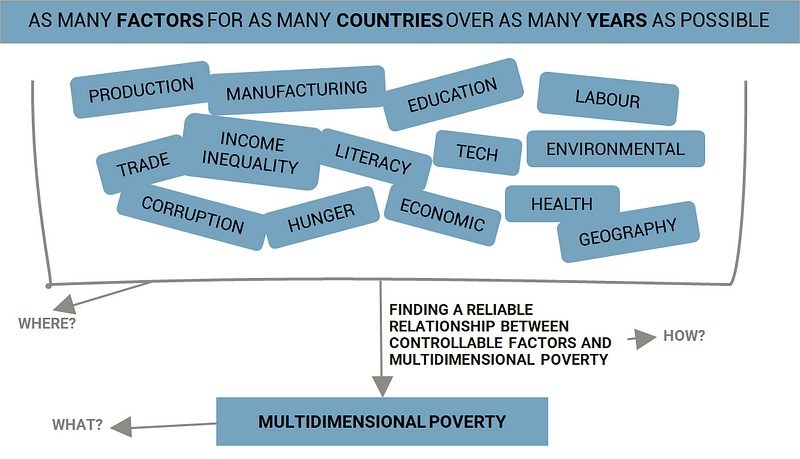
So, the idea was to take all (or as much as I could) of the macro data available out there for as many countries spanning as many years as possible, use regression models (machine learning) to isolate the 10–15 most potent factors / indicators and see if I could use machine learning again to build an inferential relationship between those isolated factors and multidimensional poverty (see above diagram). And then, when I had that relationship, to create a dashboard of sorts that could actually be used by a non-technical user.
Sounds straight-forward, right? In theory maybe, but I had to figure out a lot:
A) What the target variable for multidimensional poverty would be. As in what metric am I predicting / trying to understand?
B) Where I could find all the factors or data that impacted this poverty metric (and how to merge and clean it when I did find it).
C) How to find a reliable relationship between these controllable factors and multidimensional poverty.
D) And how to friggin’ put all that into a fancy usable dashboard.
A) Deciding The Metric To Use For Multidimensional Poverty
The UNDP has an index for multidimensional poverty called the Multidimensional Poverty Index (MPI). You’d think I would just use that, right? Yes, that was the plan. But there are not enough years of data associated with it, not enough countries covered and it’s also complicated to calculate. Scratch that.
I could use Extreme Poverty — it’s easily available with a lot of data points but then it is one dimensional, and goes against everything that I stand for. Scratch that too.
Then I came across the Human Development Index (HDI) and there was a feeling that Jesus had returned. It was multidimensional in that it considered education, healthcare and income (close enough to MPI), there were many data points associated with it across many years and countries and it was fairly easy to calculate. Yes, it did not measure poverty specifically, but it did measure well-being, and yes it isn’t a perfect metric, but let perfect not be the enemy of good.
Ta dah! Sometimes all you need is a little rationalization.
B) Finding The Data (And Cleaning It)
Next, I had to source the data from all over the world wide web. And source it I did. I had to actually stop myself at some point because this process of data gathering can be endless. In the end, I collected data from 27 data-sets from 6 different sources that spanned over 59 years across 147 countries (mas o menos) incorporating some 800 factors / features / indicators.
For Python Coders: There is a cool World Bank data wrapper you can use to do massive data pulls in Python itself. Also, you can find all the data I collected, cleaned and merged, along with the code, here (the Jupyter notebook is called “A — Merging Data”).
Sounds impressive, I know, but there was also a lot of junk in there as well — a lot (A LOT) of null values, a lot of skew, duplicate factors and factors that were highly correlated (closely related to each other). As all data scientists know, all that had to be dealt with. And it’s not always a fun process, but it’s pretty damn important.
I used Python and Excel to clean and merge the data — that wasn’t that hard. The null values however, were the cancer in my data set.
After merging and cleaning (somewhat) the data, this is what it looked like:
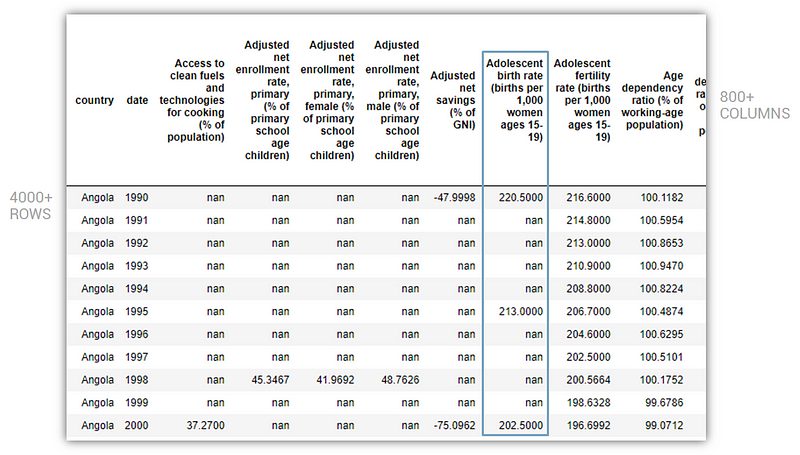
It had over 800 columns and 4000 rows but as you can see, also a lot of null values. The problem with null values is that if any row or column has a null value, it’s not friendly with machine learning. You either drop the null values (and hope that there is enough data left behind) or you try and populate it intelligently.
I had so many null values in my data set that if I dropped all null values, I would lose all my rows and columns, i.e. all my data. That’s no good.
So in order to give my project hope, I had to impute values with some logic. Thank god for Machine Learning. I used the power of machines to generate approximate values for those damn null values based on other information that was available for each country. It was a pretty complicated process, but this is what the same sample as above looked like after the imputation process:
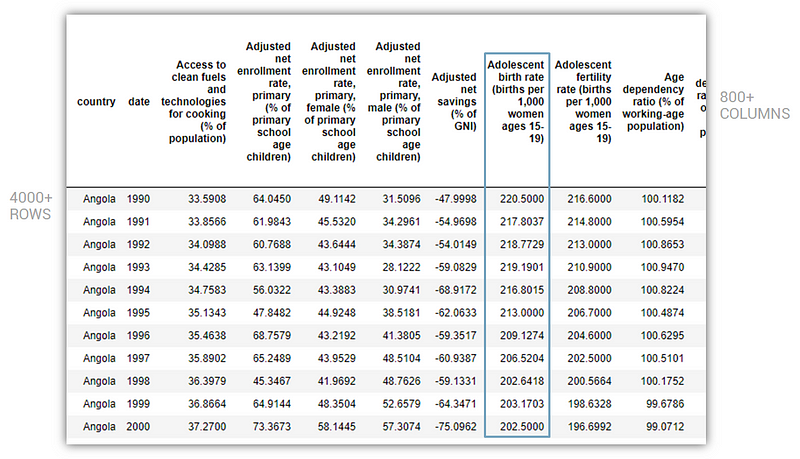
Now you’re probably thinking, “Wait, WHAT? Did you just create data out of thin air? And you’re going to use this fake data to solve poverty?”
Yes, a red flag for sure, but there is a solve for it. As long as we are using true data to measure the accuracy of our machine learning model (i.e. as the “test” set), we mitigate the risk of the imputed data leading us sideways. More on this as we proceed.
For Python Coders: Sklearn has an incredible iterative imputer that is still in its experimental stages, but for my intents and purposes, it worked flawlessly. The sklearn docs for the imputer can be found here and you can see how I used it on my Github here (it’s in the Jupyter Notebook “B —Imputing Missing Values”). For the imputation process, I had to extract data for each of the 147 countries and impute values for each country. The above file also has all the functions associated with that process.
After cleaning, merging, imputing and dropping nulls (that were not even imputable), my final dataset was a tidy 1,353 rows x 513 columns that spanned 28 years across 46 countries and had over 500 factors / features.
C) Isolating Top Factors And Finding An Optimal Relationship (Model) With Well-Being
Now that we had the data, it was time to get into the heart of data science. The first part of the process was to isolate the top 10–15 factors. Why? Because if I was going to build a dashboard for a non-coding user to use, it could not have 500 factors to toggle. That would drive the user clinically insane, but more importantly, away from my dashboard. So I had to dwindle the factors down. How?
The criteria I used to filter down these factors were threefold:
- Impactfulness (if that’s a word): How strongly correlated are the factors to well-being. For instance, is corruption more (inversely) correlated with well-being or is unemployment? I used machine learning algorithms to rank the impact of each factor.
- Controllablility: Can an entrepreneur or an individual actually impact this factor within reason? I didn’t use any fancy data science for this, just my intuition. Magic also has its limitations.
- Un-Relatedness: The factors could not be heavily correlated to HDI (the wellbeing metric) and to each other. Because if they were, that would be cheating / too easy.
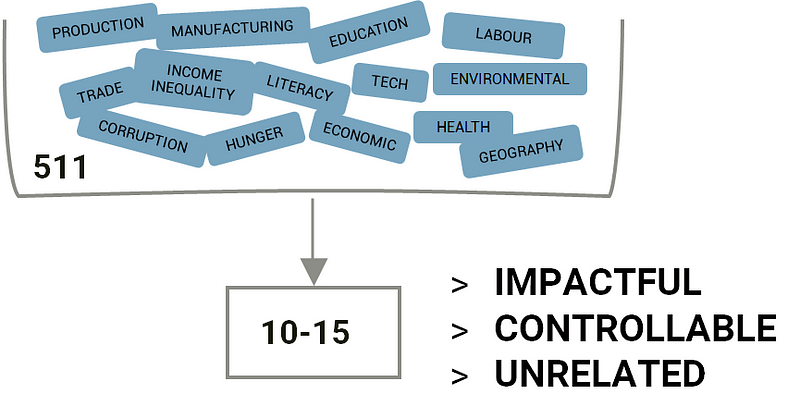
For Python Coders: To distill the impactfulness of the factors, I was restricted to using those ML algorithms that were inferential, i.e. those that either gave me coefficients or feature importance. So I couldn’t use neural nets or KNN, but I ran a hole sleuth of regressors that were inferential— Lasso, Ridge, Elastic Net, Decision Trees (piled on with AdaBoost) and Support Vector Machines.
My target variable was HDI and my X (predictor matrix) comprised all the 511 factors that we had in the dataset. My test set consisted mainly of true (non-imputed) values which mitigated the risk of the imputed data contaminating the model.
After running all the models, the best one happened to be LassoCV (which happens to be the case more often than not) which gave me a R2 score 0.94 on the test set and a score of 0.98. Since the data I was using is annual, I also ran a time-series cross validation which gave me a test score of 0.97. So, all in all, the model was looking good.
You can find this on my Github project page here (it’s in the Jupyter Notebook “C — Picking Predictors & Final Models”).
This was an super iterative process. I ran many machine learning models and each time, they surfaced a new factor or two that was either highly correlated to the components of HDI (eg. GDP is highly correlated to Gross National Income Per Capital which is used for calculating HDI) or something that was not necessarily controllable (not sure how an entrepreneur can change Land Mass unless you’re Dubai and can build islands). So, I had to drop those factors and run the models again. And again. And again.
The other issue was that 10–15 factors were too little, so in the end, after a massive Mulan-esque struggle in which I went down a black hole for a quite a while, I narrowed down the 511 factors to the following 19 factors that somewhat met the criteria I had highlighted earlier:
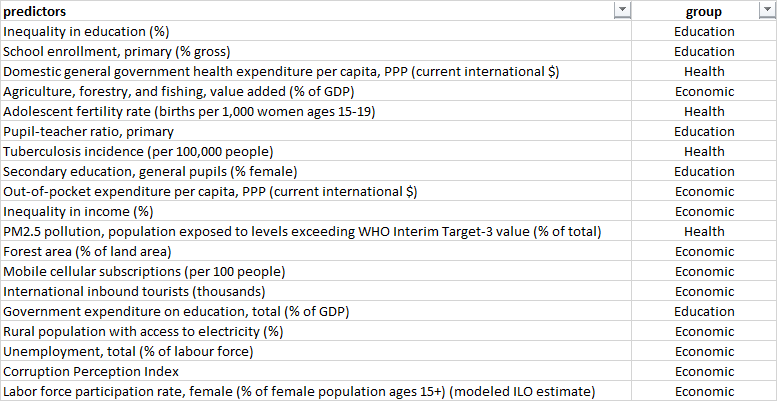
These factors / predictors are by no means perfect, and you can plug and play other factors as per your fancy, but for this exercise, these seemed workable.
Okay now on to part 2 of this step— building the final models using these 19 isolated factors.
As you are very aware by now, we are trying to predict well-being using Human Development Index (HDI) as our target variable. It’s important to understand how HDI is calculated.
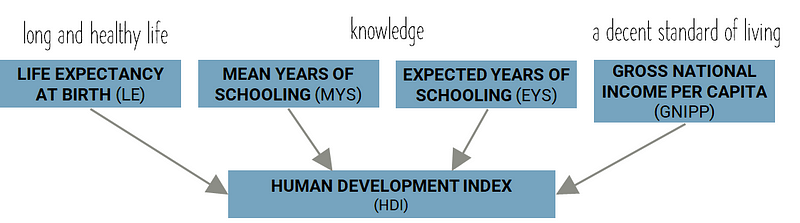
HDI is made up of four component metrics — Life Expectancy at Birth, Mean Years of Schooling, Expected Years of Schooling and Gross National Income Per Capita. There are some mildly complex formulas that are used to take these component metrics and calculate HDI, and you are more than welcome to explore that by going here.
The goal was to predict each of these component metrics and then use those predictions to calculate HDI. This would be the basis of building the final model with the isolated (“top”) factors. It would incorporate 4 mini-models for each of the component metrics that would then link to HDI. Something like this:
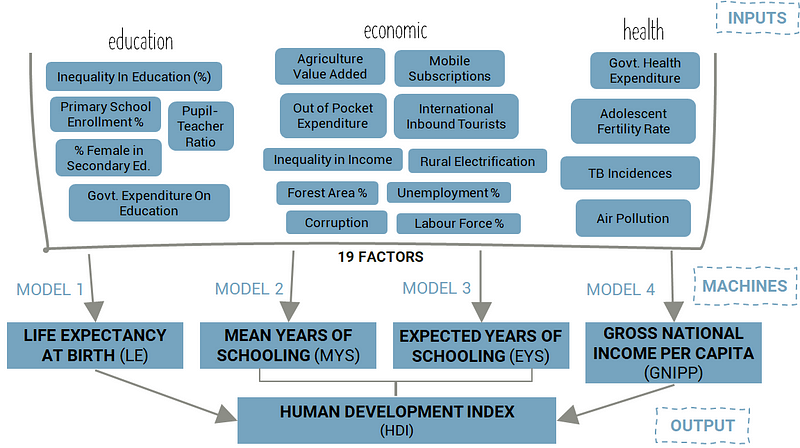
It was time to model!
For Python Coders: A guardrail here was that I could only use one type of machine learning algorithm. This is because whatever output I got, I had to be able to export it into Tableau to be able to create an interactive dashboard. Since I didn’t have Tableau Server and was using Tableau Public (FREE!!) to build the dash, I couldn’t use TabPy (a library / tool that allows you to run Python code on the fly and display visualizations in Tableau). TabPy (as of the writing of this post) only works with Tableau Server which costs $$$. Which basically meant that I could only use only linear regression models, because linear regression gives me an equation. Once I had the coefficients and intercept of this equation, I could export them into a CSV and use that to build the same linear regression equation in Tableau. Yes, this was complicated and yes, I wish TabPy worked with Tableau Public.
But you don’t give up, you adapt.
For those who are not familiar with machine learning, a common metric used to evaluate a machine learning model / algorithm is called R-squared (R2). Feel free to read more about it, but what you need to know for this post is that a R2 score of 1 means that the model is perfect and 0 means that it sucks. So we want a R2 score of something close to 1.
After building, tweaking and optimizing the machine learning models, these are what the final scores of my model looked like:
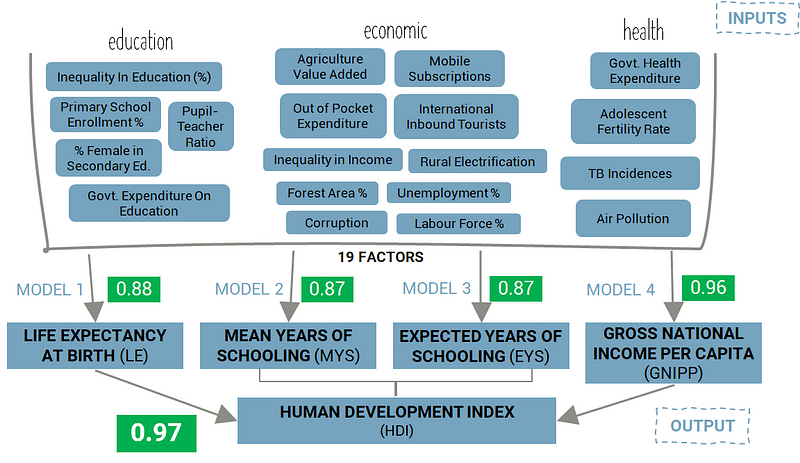
Healthy model, right? Woohoo!
If you’re wondering why the component models have lower scores than the final score for HDI, it’s because those components are used to calculate other indices which are then weighted to calculate the HDI, and in that entire process, our models fare fairly well after abstraction.
For Python Coders: The above scores in green represent scores on the test set that comprises true values only (non-imputed values). This is done to mitigate the risk of using imputed values in my training data.
I used LassoCV again for each of the four component models (Life Expectancy, Mean Years of Schooling, Expected Years of Schooling and Gross National Income Per Capita). I further optimized these models using GridSearch. I had to reduce the skewness of some of the factors / features and did so by essentially logging the highly-skewed factors. Factors were “highly-skewed” if their skew was greater than 1.
Once the four models were tuned, I used their predictions to calculate HDI as per the UNDP formula. I then ran the calculated HDI numbers against the actual HDI numbers and it gave me an R2 score of 0.97. And all in all, the score was pretty dandy.
You can find all this in more detail on my GitHub project page here (its in the Jupyter Notebook “C — Picking Predictors & Final Models”)
The model was built. The score was good. It was now time to export this model into a fancy Tableau dash.
D) Taking It All Into Tableau To Build An Interactive Dashboard
The goal of this dashboard is to put the power of machine learning into the hands of a user who doesn’t really know how to code. If I could give my target audience a dash which was somewhat intuitive to use, the machine learning model I built would be a lot more scalable.
So who is my target audience? Social entrepreneurs, primarily, and other folk that work in the impact space would be secondary.
How would this be useful to them? Maybe this would give my target audience a tool they can use to see how different controllable factors impact well-being. For instance, if they reduced corruption by 5%, what could the approximate impact on well-being be? Or if they increased rural electrification to 100%, how would their chosen country’s well-being change? All this would be based on data that spanned over 28 years across 122 countries* of all type, and it would be driven by a relationship that was calculated using machine learning that humans wouldn’t be able to do in their head (or by hand, or easily in Excel).
Obviously, this isn’t a perfect relationship. But as I mentioned earlier, it is something that they can use as a starting point for their local grassroots research. That’s how I am going to use it.
It is relatively easy to make sexy dashboards in Tableau because well, it’s a point-and-click software. But, because of that, it’s also pretty rigid. And because I used the free, public version of the software, there were some limitations on how much funky stuff I could do.
Essentially, I had to export the machine learning model from Python into a format that was digestible in Tableau. I also had to build the dashboard in such a way that I could take in information (or inputs) from the user. I wanted to give the user toggles they could toggle and see instantly how changing a factor would impact well-being.
After a lot of toil, this was the final output (that you might or might not have clicked on in the beginning). If you’ve made it all way till here in this post, I hope you do have a gander, and if you have any thoughts, don’t hesitate to share them. It’s not perfect, it’s a little clunky, but it might be useful. And if it is (or is not), let me know (or not know).
I learnt Tableau by myself — it isn’t too hard if you’re willing to give it time. There are a lot of YouTube videos that are helpful, and Tableau also themselves host a bunch of training material that might be a good place to start. You can find the working Tableau dashboard file I used on the GitHub project page.
For Python Coders / Tableau Experts: As I said earlier, I was limited by Tableau Public. If I had Tableau Server, I could have used TabPy and leveraged more complicated models. In the end though, my scores were pretty decent so I’m not fussed. The .twbx file for the dash is on the GitHub page for this project. Enjoy!
Findings
I am about to become a social entrepreneur. So I was pretty much the target audience for this dashboard. I learnt a ton. It might seem a little controversial up front, but you’ve got to realize that this is not perfect; it should merely serve to narrow the starting point for further local, grassroots research.
- Rural electrification could be a game-changer. As per the model, it seems to have highest impact on well-being. For India, if we increase rural electrification from about 77% to 100%, we could see a two point increase in overall well-being.
- Governments need to spend more on health care. This was also strongly correlated to well-being. For India, if we triple government health care spending per capita, we would increase well-being by two percentage points. This seems small but currently India spends very little per capita on its population (~$61) versus most high-income countries who spend an average of over $2,500 per capita.
- High adolescent women (15–19 years) fertility rates have a strong negative impact on well-being. This seems obvious and as expected, had a high level of importance for well-being. It’s important to reduce teenage pregnancy.
- We need to focus less on agriculture. An increase in value added by Agriculture, forestry and fishery could lead to a decrease in well-being as per the model. This might seem a little controversial, but it highlights how the world is moving away from an agrarian society towards more of a service-based one.
- Lack of sufficient primary school enrollment and inequality in education are real barriers to well-being. No surprise here, education is everything — it’s just that it takes a generation or more to impact change through this.
- Relying on governments to spend on education might not be the answer. Again, a little controversial, but it seems like government spending on education is inversely related to well-being. Note that as per the model, it is not a significant predictor so it does not impact well-being very much on the whole.
- Unemployment, labour force participation rate and inequality of income might not contribute as much to well-being as I thought they would. As per the model, these didn’t impact well-being much at all. This was a bit of a shocker for me because this is what I want to focus on. I need to reconsider how impactful working on unemployment and job-creation really is.
Limitations
As I have mentioned over and over again in this post, this is not a perfect model by any means, and it should serve, if anything as a starting point. Apologies for being a broken record, but it’s important to not make sweeping conclusions from what this model spits out. And here’s why:
- The model currently generalizes across countries and dates which can be improved with more complicated models (think Bayesian Multivariate Time Series). Along these lines, this model could suffer from Simpsons Paradox, which basically means that what is true for all countries might not be true for a specific country.
- Other factors (outside of the 19 that were isolated) could have been used to build this model with similar (but not necessarily the same) levels of accuracy.
- HDI is not a bullet proof metric to measure well-being. There have been contradicting findings around the usefulness of some of its components such as Years of Schooling and Life Expectancy.
Next Steps
Remember this upside down triangle?
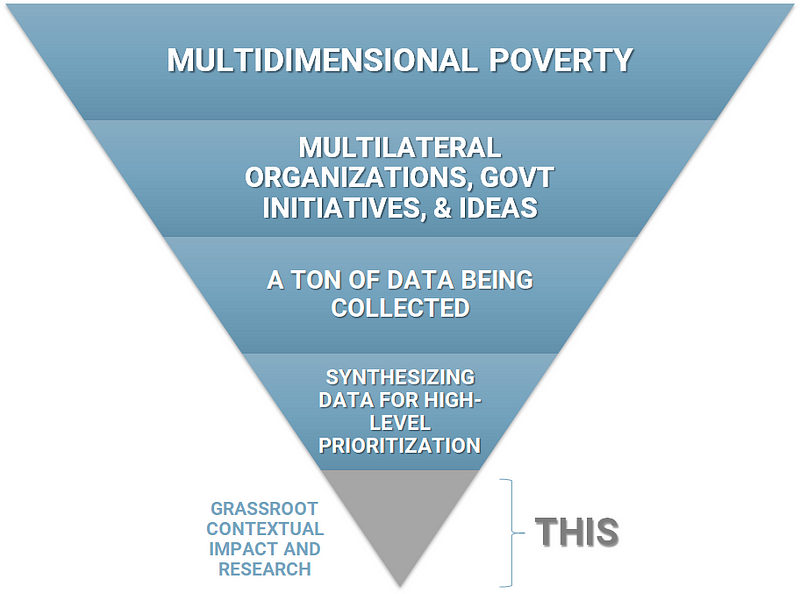
I feel I’ve given the “synthesizing data for high-level prioritization” a fair amount of time. I need to now use what I have learnt from this exercise to figure out how I am going to go about doing grassroots research here in India.
There is already a ton of that taking place in India and around the globe. This local approach is arguably the most useful and impactful, especially when it comes to deep, long-lasting change. The likes of J-PAL, IPA and Evidence Action are conducting some incredible randomized control trials that are changing the way we approach positive change-making at a local level. Many grassroots non-profits (Barefoot College, GiveDirectly) are also improving local livelihoods directly. And I must give a special shout out to Our World In Data — they are doing incredible things synthesizing data that is available at the macro level.
So here I am in India, trying to process all this. Not going to lie, I am little overwhelmed by all the information and the experiences and the books that I have consumed over the last two years. The most crippling part of this is the dangerous (but important) desire to get this as right as possible, without overthinking myself to death.
I need to see if rural electrification is as impactful as the model claims it to be. I need to reevaluate the importance of job creation and labour force improvement. What about turning waste around? It wasn’t even in the model but as consumerism increases, so does waste, and the whole jam of circular economy might start making a lot more sense. Or not.
Uncertainty isn’t fun, especially when you know that it is important to be uncertain right now.
Thank you for reading all the way till here. Seriously. Send me your email, and I’ll see how I can mail you a beer. But there might be quiz to filter out those that just scrolled to the end. Wait, let me just build a ML model to figure that out. The machines are coming.
*After condensing the factors from 511 to 19, I had more data available that did not have to be excluded by dropping null values as there were less columns that were being used.
Jagriti Yatra: The Mother Of All Roller Coasters
Okay no, so it’s not an actual roller coaster.
Jagriti Yatra is a fifteen-day 8,000-kilometer train ride that lugs over 700 people around India with the ambition of igniting their entrepreneurship spirit. The train goes from place to place, visiting local social entrepreneurs that have already dented the landscape in largely positive ways.
I couldn’t have found a more perfect set up for an introductory crash course on the impact space in India.
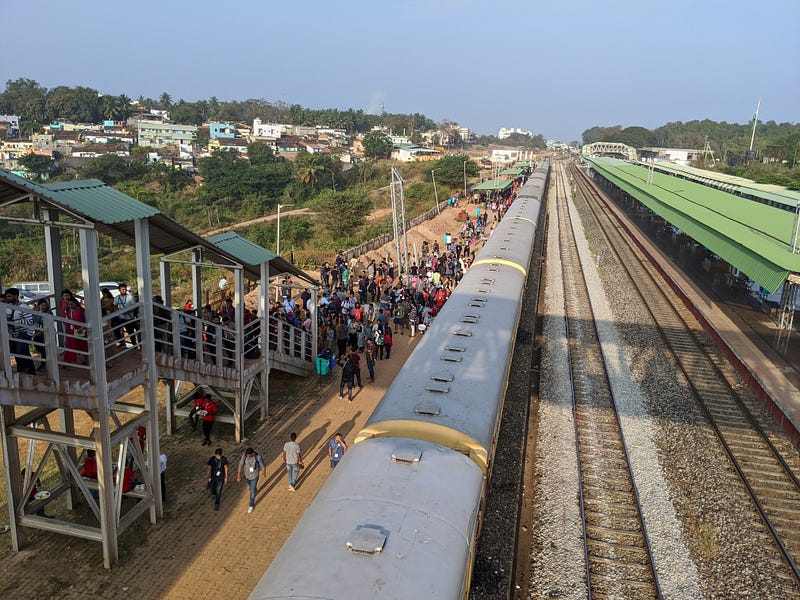
This long-tailed journey is by no means luxurious. It’s anything but. You’re squeezed into bogies that are non-AC (a traditional Indian 3-tier if you know what that is), sleeping somewhere on a triple bunk bed that averages six feet by three feet, using squat toilets and showering with buckets, all the while hoping that the water doesn’t run out as the train tries to cater to the hygiene of 700 people.
But that’s the point. It’s not supposed to be comfortable. It’s supposed to be real. Add that reality check to a supremely diverse group of Indian youth, and what emerges is a roller coaster of conversations, emotions and experiences.
So yes, Jagriti Yatra is somewhat of a roller coaster. And all-in-all, like most roller coasters, this roller coaster was a ton of fun.
Induction
For our Yatra, there were about 450 participants and about 250 support staff who were living on the train (think everyone — from the CEO to the pantry staff to the cleaners). The participants or the “Yatris” were divided into around 65 facilitators — the older, supposedly wiser crew — and about 400 younger Yatris who averaged at about 24 years of age. What united us all though was a hunger to learn more about entrepreneurship and nation-building.
Since I was slightly older and supposedly wiser, I became a “faci” — short for facilitator (but way too close to fascist). Each “faci” was assigned a cohort of six younger Yatris who we had to guide or facilitate while explicitly avoiding any form of paternalism. So far, spot on and not very fascist.
The induction process was a well-organized ball of chaos. There was flag-waving, dancing, key-note speakers, registration forms and quick health check-ups (really quick). We were also introduced to a ritual of sorts — a song and dance that everyone was supposed to partake in. And the kicker — we would be doing this ritual at every stop, whenever we could and with as much energy as possible. When I sent over a video of this to my friends, the responses averaged out to: what cult have you joined?
All cults are not bad though, right? As long as they weren’t spiking our drinks with anything.
The First Stop
Our first stop was Hubli in Karnataka. It was a day and half worth of low-priority train time from Mumbai. And since it was our first stop, I was itching to get out and see.
Each stop had a “role model” who is essentially a seasoned entrepreneur turning the tide, one wave at a time. They talked to us about the waves they had conquered, hoping to seed ideas and inspiration, especially among the youth.
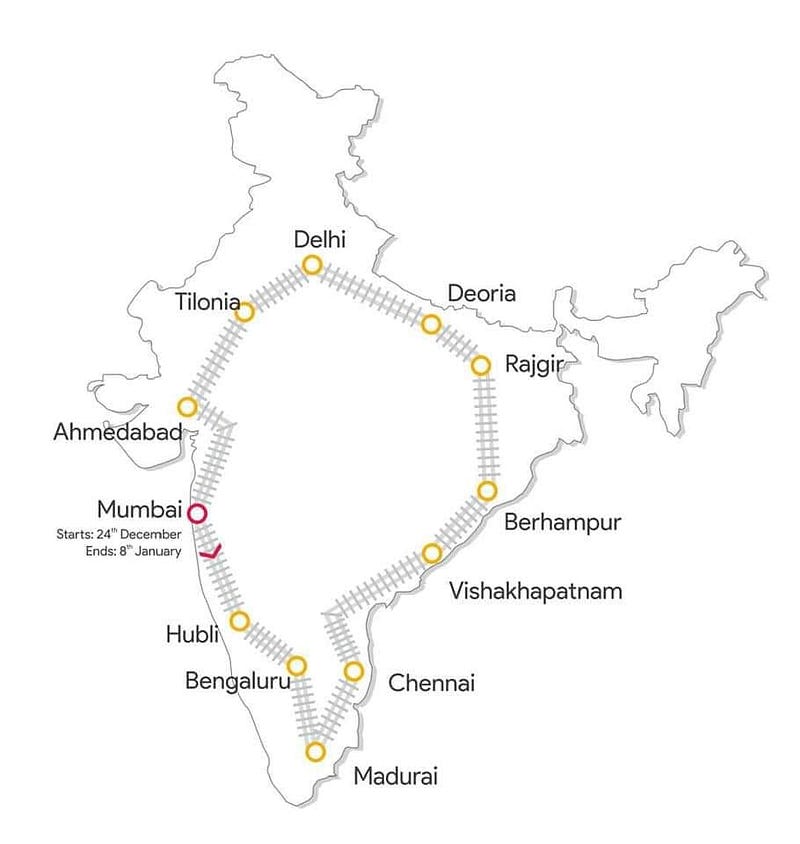
In Hubli we visited a semi-alternative school run in a village by some kind foreigners. It is a free school run on donations that provides schooling to the most in need in the village where it is. They use music, dance and art to mine minds while floating in a campus supported mainly by solar energy and trees. It was peaceful there — the kind of peace that is only extenuated by the innocent rapture of a child.
But what I quickly started to realize was that this Yatra, this journey, was less about the stops and the ‘role models’ along the way. It was more about the people living on the train.
Conversations
It’s quite the logistical nightmare to transport 700 people across 12 different places in India in 15 days on a train whilst also feeding the hive four times a day. Also, since our train was a private train, it was classified as low priority. Which meant it moved along at the fancy of the Indian Railways. Which essentially meant a lot of ambiguity. Let’s just say that uncertainty and logistics don’t go well together.
Once we arrived at a stop, we were loaded into ten buses and transported to the village or the social enterprise we were supposed to be enlightened by. Since this happened every day, it’s safe to say we spent more time on the train and in buses than at a stop. This meant that there was time for conversations with people living on the train. A lot of time. Luckily, that just happened to be the best part.
For instance, my cohort had six guys from six different states — a Kashmiri who runs a school there, a Maharashtrian with an MA in English Literature, a PhD student from Odisha, a Bengali who studied at the Aurobindo School in Pondicherry, a Hyderabadi ethical hacker and a budding entrepreneur from Delhi. Not only was there diversity, but there was also a ritualistic energy that brought us together.
And the sheer proximity of living together quickly transformed the formal exchanges into informal fraternity. We tried to have check-ins and check-outs every day as a cohort. While we weren’t always successful, we talked about everything — our goals, our feelings, our opinions. And I learnt more from them than they can imagine.
It was a safe space. Safe enough for the Kashmiri to share what he wouldn’t have had the courage to share in Kashmir. Safe enough to talk about sex and sexuality that just seems so voodoo in most of India. Safe enough to talk about all sides of a story that is gripping India right now and has ripped India apart in the past.
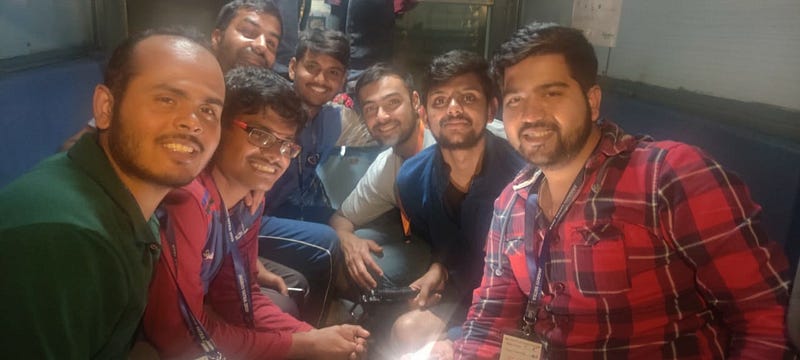
That was just my cohort in our little compartment. There was more energy exuding from compartments next to us and bogies beyond ours. And it wasn’t just the Yatris, we also had seasoned entrepreneurs, educators and thinkers from across India living on the train. They brought in a sense of sophistication and perspective that filled the rawness of the youth with colours unseen.
The nation of people on the train served my hunger to learn about India with ideas that were foreign to my foreign upbringing. They challenged my inner journey like no other, adding dimensions to my morality that only the clarity of experience can corroborate. Where else was I going to find 700 people from different parts of India from different economic backgrounds looking for purpose, looking to nation-build? Even if I did find them, how was I going to convince them to be locked in on a train for fifteen days and have conversations with me?
There was an excess of conversation potential. An excess of a potential to learn about India in a way that no book or no documentary can encompass. We were spoilt in the most wonderful of ways.
The First “Shower”
My first shower took place a couple of days in. It was a fist fight among prisoners. No, not really. But we did battle for water, buckets, mugs, permission and we were technically trapped on a train. So, yes maybe it was a passive aggressive fist fight of sorts.
Water only gets filled at certain stops, and since there are about 700 of us wrestling for it at somewhat similar times, it is a precious commodity. There are about twelve curtained compartments in a bogie upfront for about 400 men to share. You’ve got to play it smart. It needs to be a balance between how early you’re willing to get up, how cold you’re willing to feel, how long you’re willing to stand in line and when the water tanks get refilled.
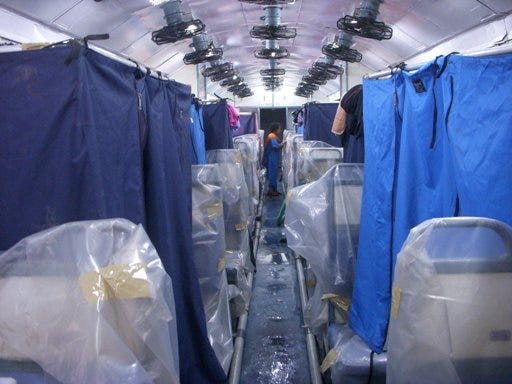
We were stuck at a station (low priority train, remember). The water was out. We were sticky and smelly after a long day out. I saw a couple of people in desperation take a bucket from the shower bogie, go to the public tap at the station, fill up their bucket and muscle their way back to steal a bath. Brilliant. I was getting a little desperate too.
I rounded up a couple of bath-hungry folks from my bogie and we looked to replicate this ingenious plan. We grabbed a bucket each and hustled towards the tap some ten bogies away. But, a twist — we were stopped by one of the organizers. I say “stopped” a little sheepishly here because apparently, we were doing something wrong. As a thirty-year-old stubborn man, I clearly didn’t like illogical restrictions. The train was at a standstill. The water was out. We were smelly. And the tap was right there. I argued (obviously, principles) but to no avail, and succumbed to an unconvincing “no is no” argument. While I was arguing for my rights, one of my accomplices sneaked across to the tap successfully, so at least someone benefited.
Upset like a little child, I huffed my way back to my bogie, contemplating schemes to sneak a bath in. Admittedly some of my schemes were probably a little too dastardly so I’ll spare you the judgment. When some sort of inner stillness was restored, we asked the bogie guard when the next water refill would be. He confided, we scheduled and then we conquered.
It was the most wondrous bucket bath I have ever had.
Never have I ever planned so extensively for a “shower”. But I also learnt that this was pretty much the norm in many hostels and parts of India. Probably with less scheming, but definitely with a lot of buckets.
A Blurring of Sorts
Our second stop was Bengaluru. They had setup a fair full of social entrepreneurs and non-profit leaders there. It was interesting but I wonder what incentive the enterprises had to be there. We weren’t looking for jobs, just for enlightenment. I’m not complaining though, it was fascinating.
We then snail-ed our way to Madurai to visit Aravind Eye Care. I was particularly excited about them because they are the epitome of a social enterprise. But we arrived horribly late, so we didn’t see any of their eye care centers, just heard some people speak. That itself was incredibly inspiring. For instance, they are currently working with Google to use machine learning to detect early onset of blindness. After their presentation, there was a panel on healthcare in India that was interesting, but I would have preferred to have been out there seeing Aravind’s world instead. And then we got stuck in Madurai at the station for way too long, because low priority, remember?
Chennai was next and we were blessed by the heavens above to get an inside glimpse of ISRO. We also spent a second in SriCity and then scrammed.
In Vizag, we got to see how Akshaya Patra delivered mid-day meals across the country. They are operational geniuses — for instance they evolved a machine that made 2,000 chapatis an hour to one that makes 60,000 chapatis an hour. 30x. Legends. But I wonder how they verify whether the meals they serve help solve hunger and malnutrition on a more permanent basis. The whole teach-a-man-how-to-fish argument.
Behrampur in Odisha was next where we visited a village amped up by Gram Vikas. Rajgir followed where we saw the peaceful Nalanda University ruins in the morning. Apparently, there were so many books in that university that the Khiljis took three months to burn it down some thousand years earlier. Post lunch, most of us took naps in the auditorium while some people spoke on stage. It was getting cold; the train wasn’t really comfortable, and we had heard enough about the vision of Jagriti Yatra and the Women Center of Excellence. A dip of sorts for sure, and the beginning of the blurring.
Deoria in Uttar Pradesh was next. We got to survey folks living in remote villages in a district that really struggles. It was probably the most insightful visit. We also did a quick build-a-business-model exercise around this where groups presented their ideas not through a PowerPoint presentation, but on chart paper instead. Unique, but you’re never going to build financial projections on a piece of paper today.
We didn’t sleep on the train that night. Instead we were squeezed into rooms in an abandoned school in Deoria. And no, nothing fancy, in fact it was worse than the trains. 15–20 of us were marshaled into rooms, many without doors, and in particularly cold weather. It seemed unnecessary but apparently the idea was to experience spending a night in a village. It didn’t all add up, but we did start appreciating the train more after.
Delhi was next, and the blurring was pretty much in full flow. I remember I had to go back to the Yatri Guide to recall where we had been, and where we had seen what. I’ll attribute that to a lack of proper sleep. Most people in the train were now coughing — a combination of cold weather and human proximity on the train I reckon. We still managed to sleep through a lot of that though. Humans adapt pretty damn quickly.
In Delhi, we met Anshu Gupta from Goonj. Goonj is doing some incredible work and Anshu is a passionate orator. He lit a fire up a lot of the Yatris who weren’t as sold on the importance of making an impact which was admirable. My fire has been lit for a while, so I was a little put off by the alarmism that Anshu employed. Effective, but not my cup of tea.
A lot of the Yatris that lived in Delhi took this opportunity to sneak out and get a proper shower in. This was controversial because this wasn’t technically allowed. Discipline oh discipline.
Discipline
An important tenet of the Yatra is discipline. Transporting 450 participants in a train at breakneck speed is not easy. Rules and discipline are key. Makes sense. One of the rules is that you’re not allowed to leave the train or any of the premises that we visit. Makes sense.
But maybe, a gross interpretation of this rule begs a debate? For instance, I was not allowed to go get a coffee from a canteen that was 20 meters away and within the premises of where we were. As a thirty-year old adult, this really rubbed me the wrong way. I obviously didn’t listen because principles. But then I was threatened of being deboarded off the train. And I was also asked to write a formal letter of apology. I did laugh out loud at this (and obviously didn’t write the letter), which really didn’t help my case. Yes, at one point, I was guilty of being rude. I owned up to that and apologized for it. But principally, I did feel this was a bit ridiculous. Versions of this happened to me more than once, and I wasn’t the only one. At least I was willing to put up a fight, most just sneaked out instead.
This obviously led to a lot of internal debate which I externalized pretty quickly. The other side of the debate is that exceptions are costly, so a strict implementation of the rules is important. Easing the rules or allowing exceptions leads to a bad precedence, and chaos could ensue. Fundamentally, I agree with this.
Where I was stumped was with the target demography. The 450 participants averaged to around 25 years of age, and were selected from over 2,500 applicants, and were touted to be a representation of the best of India. The question that I struggled with was that if these are the best young adults of India, can they not be trusted to take a few minutes out to go get a coffee? Can they not be trusted to make a quick visit home to take a shower while they are in the vicinity? Is that too much to ask?
I did ask these questions to other people on the train. And this is where it gets complicated. The Yatra takes responsibility for people on the train. One bad apple does spoil the bunch and could have a terrible impact on the reputation of the Yatra. Also, people from different backgrounds have different levels of freedom. For instance, younger women from more traditional homes only barely get permission to go out on a fifteen-day journey by themselves. Freedom could be a different concept for them. Their parents could be a lot more particular about how strict the rules at the Yatra are.
But then the alarm that go off in my head is are we solving for exceptions? Is that even pertinent, especially when people are sneaking out anyway? And this, quite dramatically, leads me to think about the general impact of paternalism in India. Does excessive amount of paternalism come in the way of maturity? Does it delay it? Does it encourage rebellion?
The answer as always lies somewhere in the middle. I think with the Yatra, a middle ground could be that if someone wants to leave for a few, they must fill a quick liability release form. This lifts the burden off the organizers and puts some physical toll on the participant, while maintaining the illusion of freedom. But yes, rules are important, and their strict implementation is needed to avoid chaos.
Also, it was interesting to see how the 65 facilitators broadly auto divided themselves into two different camps of thought around this. One side seemed more pro-independence and the other side more pro-rules. Less control versus more control. Bear the discomfort versus fix the discomfort. I skewed more towards the “less-control” folks, but my upbringing isn’t necessarily the norm.
I need to read up more on the more existential question of the impact of excessive paternalism on maturity. The key word here is excessive. A balance is what always seems to be the answer — just the right amount of paternalism, maybe? What’s the “right” amount though? Haha now we tread on realms of infinite regress.
The Bunker
After Delhi, Tiloniya in Rajasthan was next. That’s where Barefoot College was born along with the legend of Bunker Roy. After walking around the solar-powered campus, we were treated to a session with the legend himself.
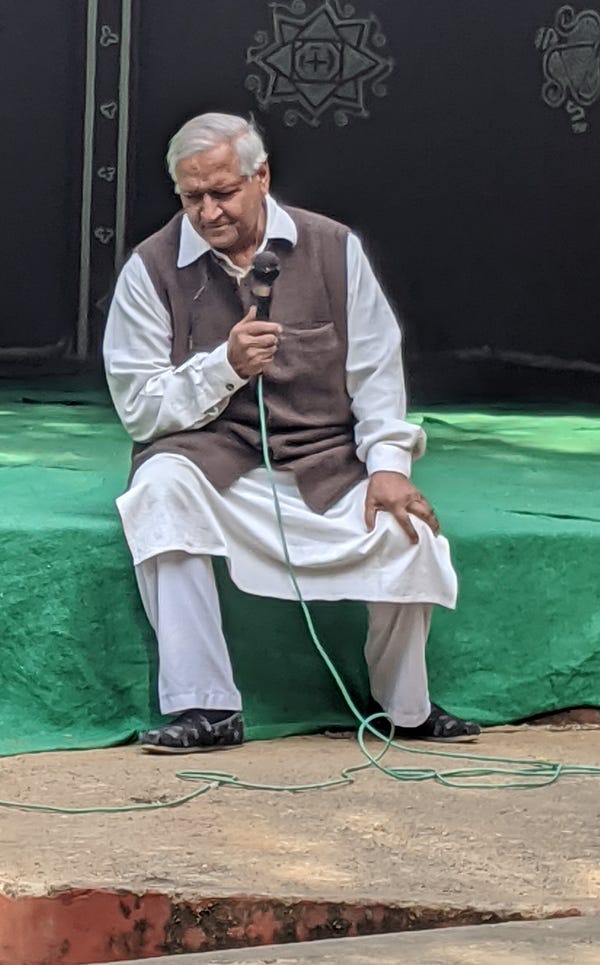
Bunker Roy appeared, sat down on stage and threw the floor open for questions. It was all a little mystical in the most wondrous of ways, and this is coming from someone who doesn’t believe in magic. He championed a level of depth that only comes from having skin in the game. Some real deep skin in the game. The wisdom flew off his tongue as easily as his demeanour. As gently as his voice. As compellingly as his presence.
Start small, he said. Do more and study less, he said. Be where there needs to be work done, not locked away in an office in some skyscraper, he said. All the while he embodied the aura that natural leaders exude — an aura not restricted by norms, but instead empowered by the confidence to reshape them. There was a sense of authenticity that no one can pretend to put on. This was him through and through. He had already walked his talk.
And I sat there, a little teary eyed, yearning to be a version of him.
At Peace
Ahmedabad was our last stop. There was an element of lethargy in the air. We were a lot more comfortable with each other and the conversations were a little less exciting. I was looking forward to a nice hot shower and a warm bed free from vibrations that was larger than six feet by three feet.
The rules became a little lax. There would be no roll call in the train that night. A little less than half the participants were going home after the graduation ceremony. Don’t know what we were graduating from, but there was a formal closing ceremony later on that was nice.
We were at the Gandhi Ashram, sitting at the edge of the Sabarmati river. I felt at peace. Familiar faces all around. Photos being taken out of a desperation to preserve the current bliss. Conversations getting personal and more relaxed. Silence becoming comfortable. It was as if all the ingredients were in place for a perfect reflection.
I came to the Yatra with two distinct goals. One was to get to know India a little better and the other — more personal, more daunting — was to see if I could fit in as an Indian among Indians. I hadn’t lived in India in over twenty years. Yes, I visited regularly, but I was defined by a different set of borders of which the Indian subcontinent was just a subset. I was someone who has been everywhere but is from nowhere, and all of a sudden, I was craving belonging.
I did get to know India better, but it wasn’t by visiting the different places, it was mainly by the people on the train. People who came from all parts of India, from all types of religions, with different languages and different economic backgrounds, and from the conversations we shared. There was common ground to be found in reason, in purpose, but it stemmed from varying perspectives and contexts.
I got exposed to a different form of thinking. A form of thinking that forms the bedrock of Indian culture which is often marred by convenient interpretations. But that doesn’t mean that the Vedic life is inapplicable today.
I embraced my Indianness more than ever. For once, eating your heart out with your hands and licking your fingers after was the norm. It didn’t matter that many of us got our Vs and Ws mixed up when we enunciated, we still understood each other. Claps were doled out like currency, a worthy cheap form of positive expression. And we Indians love acronyms way too much — wouldn’t mind if that changed.
I also learnt that reaching a conclusion after every argument is not always necessary. Sometimes it is the depth of discussion that matters more, the nuances. There was a panel on women empowerment which when asked about discrimination against women, said that they were not discriminated against — in fact, as women entrepreneurs, they felt they had a slight advantage.
Then there is the other side to the women rights movement where certain laws treat women differently than men in India. For instance, a man can serve up to three years in jail if he gets convicted for sexual harassment but there is no such explicit law made for women. There’s obviously good reason for this, but it also does portray an unneeded disparity. Someone in my cohort bought this up — “I don’t sexually harass women but why are they protected by law and I’m not? Equality goes both ways.”
There was also the voodoo topic of sexuality and sex. There is no proper sex education in India, and sex is considered such a precious, restricted and sacred commodity. I can’t help but think how sexual repression correlates to abuse. It’s not an excuse, but it could be a factor.
I got on the train while the country was in the middle of extensive CAA protests. I was expecting intense amounts of passion on the train around this, but it was a lot calmer than I expected. There seemed to be both anti and pro sentiments, but none with the amount of gusto I see on social media or among people who identify as Indians but who live outside of India. Either it was fear that censored their passion, or it just wasn’t as important to the folks as it seemed from the outside. I think it’s the latter.
I know I live in a privileged, left-wing bubble, and this experience further cemented that as true. Problems and priorities are different for people with different privileges. And when you’re still taking bucket baths or practicing open defecation, a law that directly impacts 0.003% of the population might not matter as much.
The people I got closest to on the Yatra called me ‘Angrez’ (“English”). That was almost like a violent attack on a deep-rooted insecurity around my Indian-ness, but it turned out to be a term of endearment. I blended in soon enough, leveraging humility and humour to find common ground, and accepted what needed to be accepted — I am different. Just like everyone else is in their own way. So obvious. So simple. So cliched.
I don’t think I would do this a second time, but the first time was well worth it. It pushed me in all directions, from discomfort to comfort. From embarrassingly broken Hindi to my supposedly intellectual English. From uneasy living to easy conversations. From zero to hero to zero. It was a friggin’ incredible roller coaster. And the best part about it was that there was no real safety belt.
Nairobi & Beyond
“This doesn’t normally happen around here,” My new boss explained, a little worried.
It was the first day at my apprenticeship in Nairobi, Kenya. The police had tear-bombed a mob in the busy slum of Kawangware where our office was located. The rumour was that someone had been shot, so the mob had circled around there, essentially sectioning off the cops from getting access. So, as any rational law enforcement body would do, the police tear-bombed the area to disperse the crowd.
There was a lot of scampering around us. The braver ones in our tiny office rushed over to the window to get a glimpse of the commotion. The tear gas only scarcely made it into our room as we were on the second floor. It was enough to get our eyes all misty, but not enough to have to pull them out of our sockets.
Nairobi was supposedly my last stop before I headed to India. But right now, I am in a coffee shop in London. Not quite India, but the ‘plan’ is still in place. I’ll get to it.
After over a year in Guatemala, nine months longer than ‘planned’, I knew that my stint in East Africa had to be a little more constrained – no more than six months. If the goal is to start something in India, I can’t just keep frolicking around the globe, delaying the inevitable. But working in Sub-Saharan Africa seemed like an important step in this custom MBA of mine.
Shoddy as this sounds, the income per capita, the infant mortality rates, and disease-inflicted deaths in parts of Africa are among the gravest. Add that to the exploding youth population, the lack of jobs, and the rising temperatures, and what the continent is drifting towards is a largely young population of two billion by 2050, struggling to find employment as the world boils over. There could be a lot of other things that boil over as well.
But don’t get me wrong – there has been a ton of progress over the last few decades. Overall, as much as we shouldn’t generalize, African countries are freer and more developed than they have ever been. There has been innovation and expression, a better quality of life, and a Twitterverse exploding with opinion. It’s better, but there is still a long way to go. And that’s why there is a lot of game-changing work being done there, especially in the social sector. So, I had to work there. This time I was looking for more depth – i.e. working more closely with a couple of entrepreneurs, rather than at a more superficial level with many entrepreneurs, which was the case in Guatemala.
A random spam-ish email landed in my inbox that talked about a certain Amani Institute. It was new but what I liked about a program they offered was that it involved a four-month apprenticeship in Nairobi with local social enterprises / NGOs. It also promised just enough coursework in some of the softer skills that I had been craving, but wouldn’t necessarily stretch for – leadership, management – some of the soft stuff that had haunted me towards the end of my stint in New York. Bingo. I applied and soon thereafter, I was on my way to Nairobi as an Amani Fellow. I would have gone either way, but I was glad to go there with some structure.
The six months in Nairobi were intense. We worked for three days a week and had classes three days a week. The Sunday that was left hanging, left us fellows only wanting to hang around and do nothing. I was apprenticing with a social enterprise that was trying to fight poverty through job creation in the urban slums of Kenya – yes, the one I was tear-bombed at on the first day. More importantly, they are trying to do similar things to what I want to do in India. So, the learning curve was as steep and healthy as any wealthy education, if not more. They did a lot of things well, but where they struggled was in finding operational prowess. Sadly, in the impact space, this is common.
While there is a ton of passion and drive in the social sector, there aren’t enough seasoned operators or technical folk. Add all that to the meagre pay, and what you’re left with are inefficient organizations with tiny technical expertise and gigantic hearts. Burn out is more common than you’d imagine and it happens without any splurging compensation that at least some of the corporate burnouts can fall back on.
The six months whizzed by. But it felt like I had been there an eternity, making new relationships and adapting to a new culture. It's strange how time loses it structure as we sway from one perspective to the next. I left Nairobi more ready than ever. I felt both validated and enlightened. I might have been extorted by bad cops with a gun in broad daylight, but I acquired a deep experience that has geared me up for my next steps. I want to champion the change I want to see in the impact space – this asymmetry of incentives, operational weakness and a lack of scalability and sustainability.
But I have also learnt that this is going to be hard. Starting a successful for-profit is in itself a probabilistic minority. Add the cost of impact to that and what I have signed up for becomes exponentially harder. But that’s okay. I am not doing this because it’s supposed to be easy. I am fortunate to not have to worry about going hungry, and morally, it’s hard to argue against marrying what I should do and what I want to do. For the last two years, I have been closing the gap between the moral ‘ought’ and my internal want, and surprise surprise, I have never been happier, more content, more focused and more determined. The honeymoon phase should have passed by now, so what has remained is clarity that only consistency can fuel.
So, what am I doing in London? Remember the lack of operational prowess and technical expertise in the impact space I was talking about? Well, I don’t want to be that cliché or at least that is my current rationalization. Yes, I can sing some decent finance tunes, but that might not be enough. One thing I realized in Nairobi is that I know enough about working in the impact space to start something on my own, but what I might be missing is some more technicality. I found this immersive Data Science course that General Assembly offers in London. It’s a twelve-week bootcamp that tries to download at rapid speed everything it can about Machine Learning and Data Science. Magically, I had twelve weeks to spare, so here I am. I am halfway through the course, and I feel like I have opened a new box of possibilities that is so empowering. I am not going to be a Data Scientist, but I want to use Data Science to make better decisions – to do better research, better monitoring and evaluation, and most importantly, to better allocate resources to solving this shitshow of a problem that is poverty. And as a bonus, I get to spend three months living with my best friend. When else am I going to get a chance to do that at this age?
It’s not all hunky dory though. One of my mentors called me out on this – Anish, are you just delaying going to India and starting something? While he makes a fair point, it’s only three months and I am not technically delaying when I go to India. According to the ‘plan’, 2020 was when I was supposed to be in India, and my tickets are already booked. I reach India on the 21st of December, 2019. I am excited, so friggin' excited.
But I am also scared. There is this massive cloud of uncertainty hovering around – what will I be exactly doing in India? When will I find out? When will there be some stability? And here’s the kicker, this current uncertainty is essential. If I knew it all, there would be a high probability that I was delusional, or at least being naïve. I am going to be spending the first year in India researching, prototyping and validating different solutions that holistically address the problem. I can’t rush this but at the same time I can’t take forever. This is a long-term game that I am playing and that implies unrushed progression while always finding the right range of balance between the extremes. Delayed gratification is the secret to life, however hard it sometimes is to not give into immediate temptation.
At the same time, maybe, I am attaching myself to this idea that I am going to find the ideal solution. That’s not smart either – I might be setting myself up for cognitive hell. So, I am trying to focus on starting small. I am trying to disconnect from building up something that might be too idealistic to process. I am trying to train my morality to account for all the distractions that might scuttle me. And the best part about this is that this is exactly what I want to be trying to do.
Nairobi played its part like a perfectly written textbook for what I wanted to learn. I added some beautiful people to my network of love. I also felt the bitter sting of immorality, hoping to come out stronger. I might have been tear-bombed and extorted, but I also danced on the beaches of where nature might be as wild as we can imagine. For all that and more, I am only better, yet aware that there is still a long way to go.